Work
Developing an Intelligent Gas Grid to reduce carbon emissions
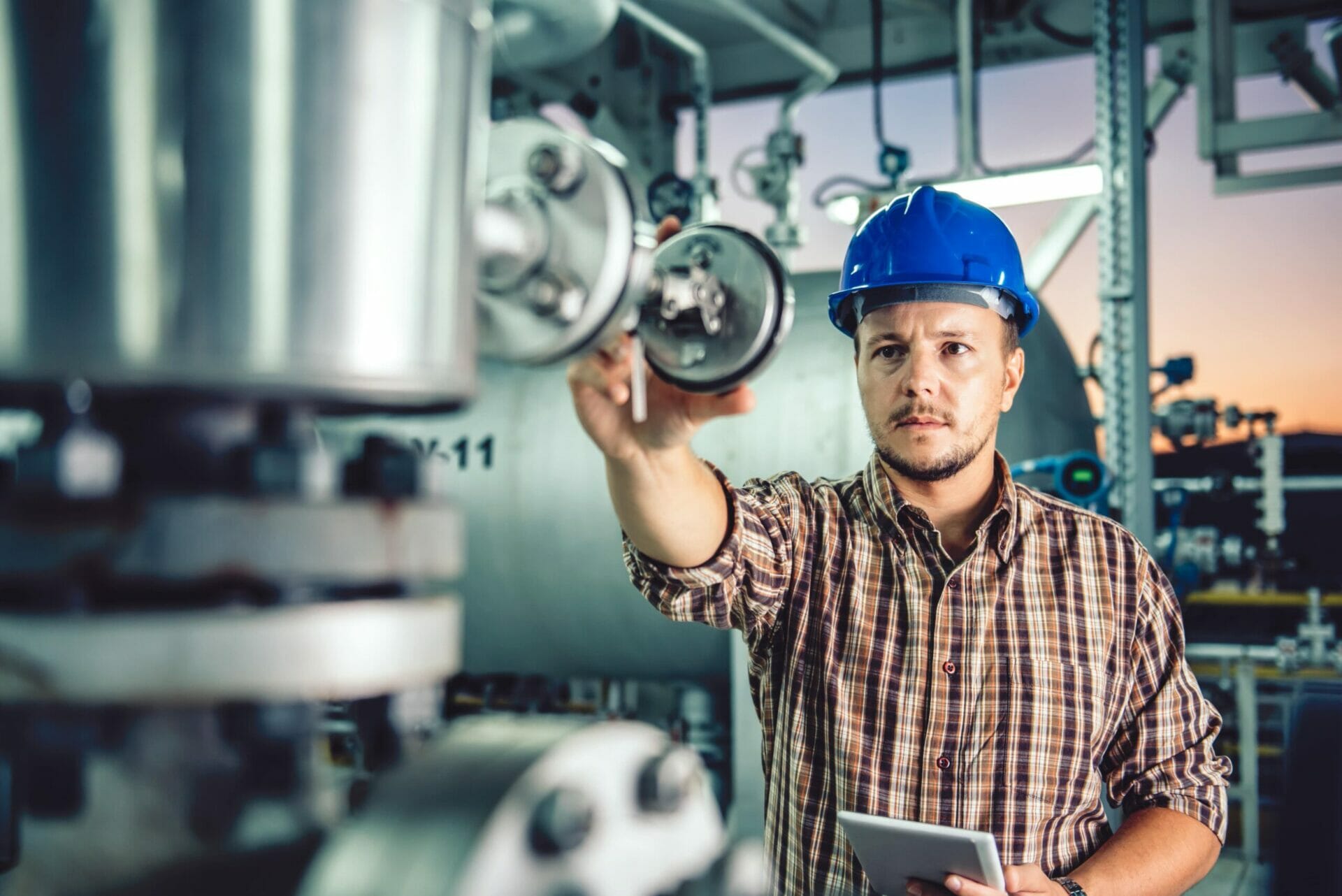
Customer
SGN Gas Distribution Network
Challenge
SGN sought to investigate the use of remote pressure control to improve network outcomes such as methane leakage, and prepare of renewable injection.
Solution
We worked as a consortium with SGN and pressure control specialist, Utonomy, on innovation project funded by Ofgem under the Strategic Innovation Fund (SIF) £450m programme. We designed and evaluated AI solutions for eight opportunity areas driven by automated pressure management and underpin the development of an ‘Intelligent Gas Grid’.
Impact
Our technical designs have been recognised by our partners, Ofgem and Innovate UK as innovative solutions to enhancing network management activities, lowering costs to consumers, and decarbonising the energy supply. For instance, two solutions showed how using AI could reduce passive methane leakage from the network and increase the amount of renewable gas feed-in.