Blog
Powering data science with design thinking
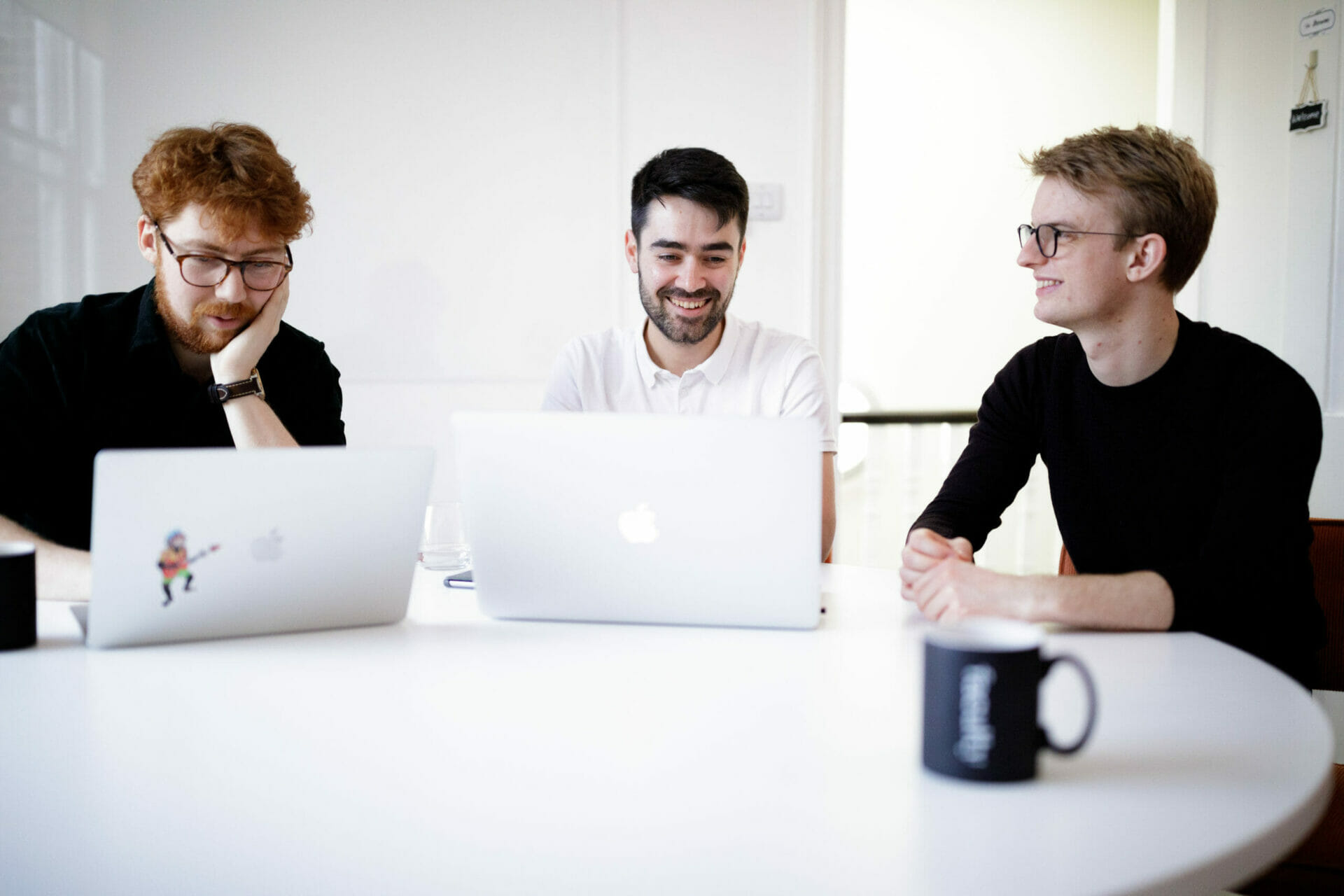
Design and data science might not immediately seem like natural companions; one is associated with art and creativity while the other is a rigorous, quantitative discipline.
As a designer at Faculty, I’m fortunate to be at the intersection of these two really exciting fields. It has given me a unique perspective into how they contrast, but perhaps even more importantly, where they overlap and how they can work together.
The principles of the design process, now widely known as ‘design thinking’, can help get the most out of data science, by providing a methodology for dealing with the ambiguous and complex nature of the challenge. Human-centred design can also help ensure the resulting insights are actionable and valuable for stakeholders, who remain at the core of the process.
The challenges with running data science projects
In any project, the natural temptation is to start by evaluating the available resources. In the case of analytics projects, that means exploring the data, slicing and dicing it in various ways, in the hope that we can come across some meaningful insights. Similarly, when tasked with designing a new product or service, we might be inclined to start with the existing solutions, instead of understanding who would benefit from the new solution, how they would use it, or why it’s needed.
The difficulty with this approach, particularly in the context of data science, stems from rushing to find answers when we don’t even know the questions we are asking. Rabbit holes and dead ends are inevitable and, even when new information emerges from the data, it’s difficult to know how to tie it back to the project brief, whether it has met the goals and how useful it really is for stakeholders.
Introducing design thinking
Design thinking has emerged as a methodology for dealing with ambiguous, ill-defined problems, by utilising elements from the designer’s toolkit such as empathy and experimentation, to arrive at innovative solutions. Made popular by design firm IDEO, the design thinking framework follows six phases (empathise, define, ideate, prototype, test, and implement) in an iterative, human-centric process.
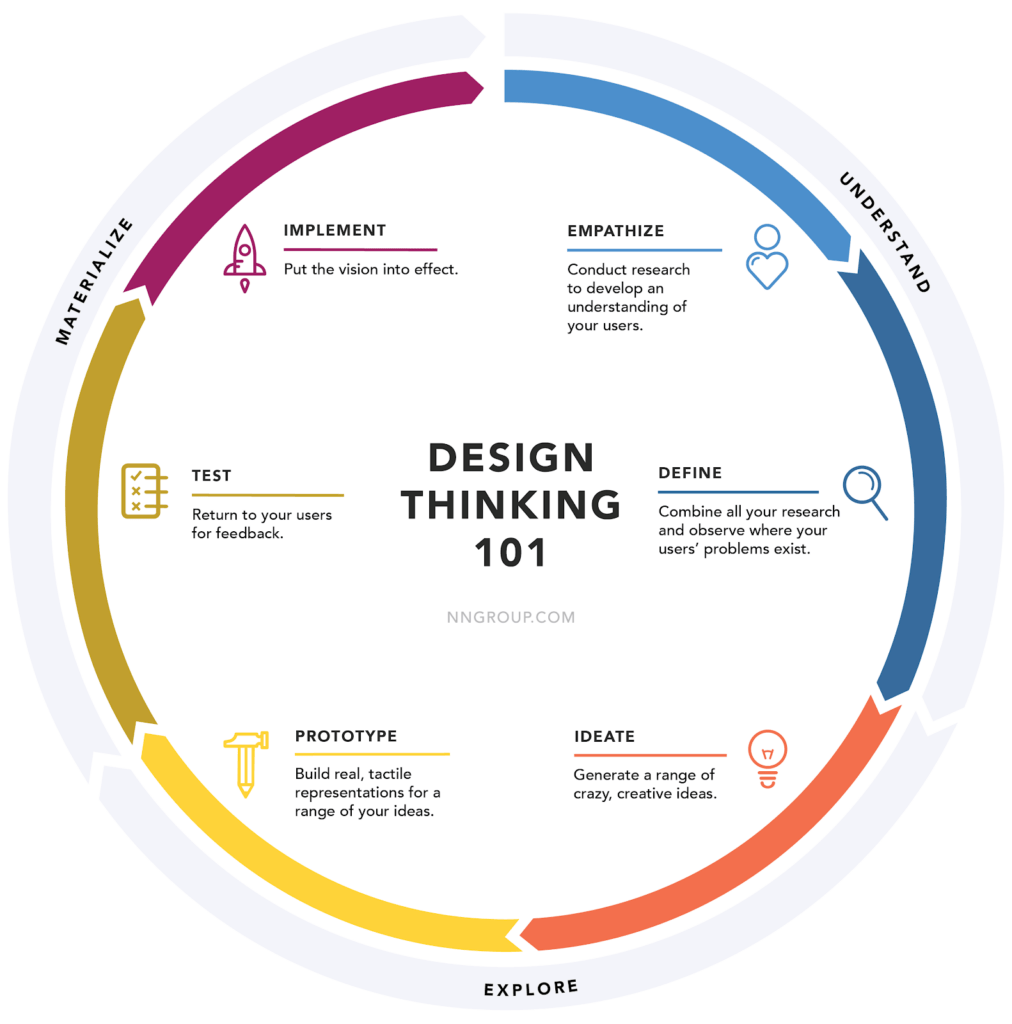
The real power in this method, I have found, is that it provides a gradual, step-by-step framework to get from a problem space with many unknowns to a well-defined solution, with increasing levels of confidence and refinement along the way.
Useful design principles to apply in data science
‘Human-centric’ data science. Remember that humans are ultimately the beneficiaries of any insight derived from data. Take the time to understand your stakeholders, what burning questions they are trying to answer, what goals they have, and what frustrations burden them. What type of actions and decisions do they need to take? Who do those impact? Do your homework (also known as ‘user research’ in the design world).
Framing the problem. ‘There is nothing more frustrating than coming up with the right answer to the wrong question.’ ― Tim Brown, Change by Design: How Design Thinking Transforms Organisations and Inspires Innovation. Designers are conditioned to stay away from the solution space until they know they have the right problem statement and they understand the domain well. Brainstorm and then narrow down a well-defined list of hypotheses or questions you are looking to answer with the data. Validate and prioritise that list with stakeholders.
Diverging and converging. Google offers a helpful ‘triple diamond’ to illustrate the design process. Because we are heavily biased by the status quo and our assumptions about the domain space, we need to force ourselves to think divergently, without constraints, at certain points. For example, throw as many ideas for possible solutions on the table before committing yourself to one. It’s unlikely that the first one that came to mind is the best and we tend to overemphasise technical limitations and therefore eliminate great potential solutions from the get-go. After diverging of course, it’s also time to converge to a specific problem or an agreed solution, that incorporates elements from ideas as well as requirements from technical and business feasibility.
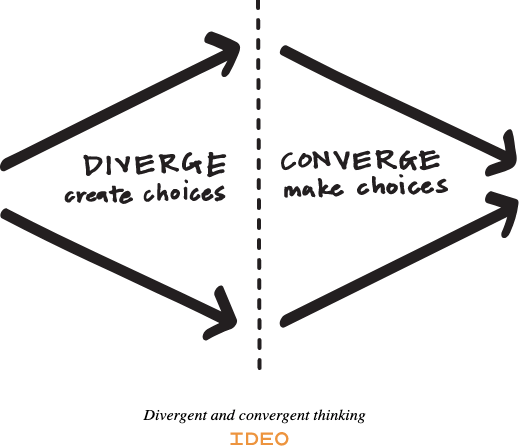
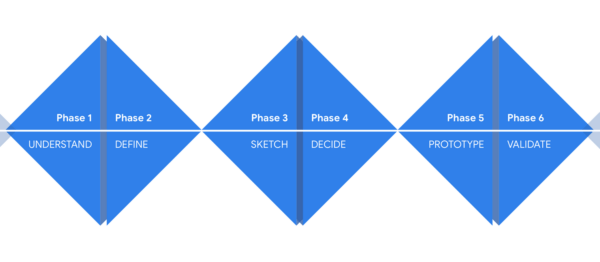
Rapid visual prototyping. It is easier to respond to things we can see and interact with rather than when we talk about concepts and ideas. A simple chart can go a long way in eliciting feedback and getting stakeholders to think about what insights matter to them. The sooner and more frequent, the better!
Explanatory, not exploratory, solutions. Having more tools and options at your disposal is not necessarily better. In fact, it’s proven to make for worse user experiences. When generating a report or dashboard for your stakeholders to view and interact with the data, aim to create a story they can be gently guided through. Help them uncover the most surprising or interesting insights by presenting them at the right points. Allowing them to explore in every direction is likely to result in confusion, a feeling of being overwhelmed, and more hard work for them.
Bring a designer in the room. Having a design thinking expert involved throughout the project will mean you can make the most out of applying these principles to data science. Give them a seat at the table!
If you’re interested in blending design thinking with data science and AI, have a look at our current vacancies here or get in touch!