Blog
Generative AI: where are we now and where are we heading?
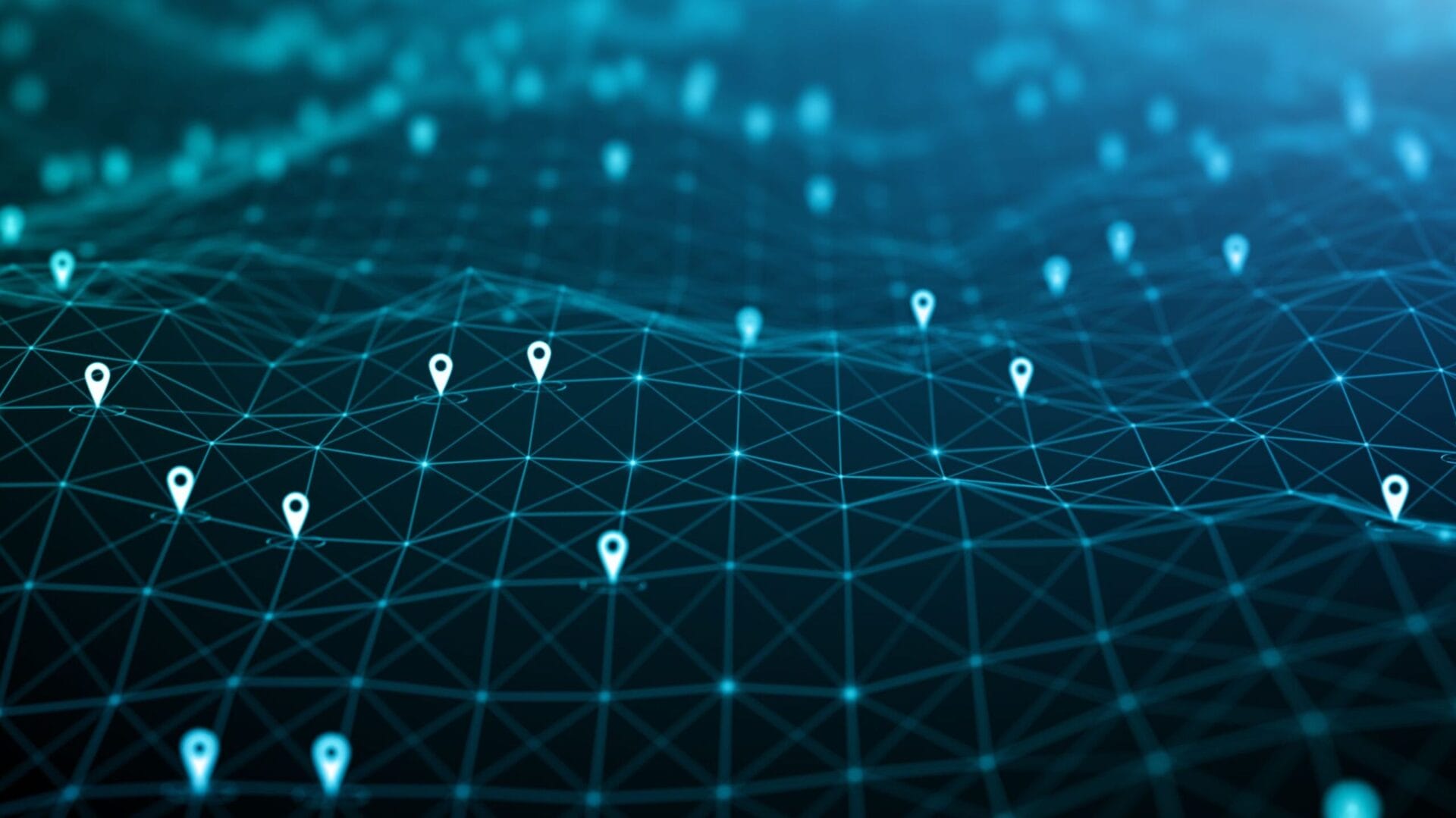
Generative AI has revolutionised the digital landscape, resulting in a surge in technological adoption across several sectors. In this article, we’ll explore how the emergence of ChatGPT rivals and the rise of more accessible and efficient models marks the next phase in technological democratisation, offering businesses enhanced opportunities as we progress into 2024.
Generative artificial intelligence (GenAI) exploded into public consciousness in 2022, swiftly gaining traction across all major industries. Within two months of its launch, it amassed 100 million monthly active users, making it the fastest-growing consumer application in history, according to a UBS study.
Early adopters range from the legal sector, which successfully used GenAI to boost the productivity of junior analysts, to furniture manufacturers like Ikea, which implemented it to improve the customer experience in its call centres.
ChatGPT: from prototype to viral phenomenon
The proliferation of generative AI was triggered by OpenAI’s release of its chatbot, ChatGPT, in November 2022. The Large Language Model (LLM) that provides the backbone for ChatGPT is the culmination of a large transformer model and a neat training trick incorporating humans-in-the-loop to ensure more human-like responses.
OpenAI continued to ride the wave of success in 2023, releasing DALL-E-3, a state-of-the art image generation model, and GPT-4, the most advanced generative multimodal model to date. Additional proprietary LLM-based chatbots, such as Claude, were released by fellow startup Anthropic.
By the end of 2023, the tech incumbents, who were previously lagging, began to release competitive models, with Google releasing Gemini Pro, which is reported to perform on the same level as GPT-4. Meta meanwhile focused on the development of generative audio models, with the open source community leading the democratisation of generative text models via huggingface, crucial for widespread adoption of GenAI.
In light of GenAI’s rapid progression to date, it raises the question: Can we expect this pace of growth to continue, and if so, what are the implications for businesses and what actions do they need to take?
GPT-4 alternative will expand business opportunities
The pace of development looks to remain high, with Google set to release its most powerful large multi-modal model (LMM), Gemini Ultra soon. It is thought that Gemini Ultra will provide businesses with competitive alternatives to GPT-4.
Indeed, as we move further into 2024, we may start to see the emergence of additional GPT-4 beaters. The release of smaller, better, and more tailored models will likely contribute to knocking GPT-4 off its perch; for example, Mistral/Mixtral, has been shown to be a fraction of the size and comparable in areas of semantic understanding.
What lies beyond Azure?
Due to OpenAI’s relationship with Microsoft, companies would have to use Azure-based services in 2023 to take advantage of privately hosted state-of-the-art LLM performance. In 2024, with the release of competitive models from Google, businesses can look to develop on alternatives such as the Google Cloud Platform. Models from Anthropic are already on Amazon Web Services (AWS), but the further development and release of competitive models to GPT-4 will open the door to companies that do not natively use Azure, further increasing the widespread use of generative AI.
Moreover, AWS recently released AWS Q, a template for businesses to create generative AI-powered assistants tailored to businesses. AWS Q, with enterprise-level GPT-4 beaters, will reduce the barrier to entry for smaller businesses to work with state-of-the art GenAI in a secure and private manner, potentially opening the door for startups in the defence and medical sectors.
Open source collaboration could lead to more efficient GenAI models
The issue of proprietary versus open source is another hot topic in the AI community.
There is no one solution that fits all. Both approaches have their advantages and drawbacks. Choosing which is the best fit for your business depends on a number of factors. For instance, proprietary models are believed to provide more security and reliability, and they work well in certain situations, such as:
- Quickly validating proof of concepts (e.g., via the OpenAI playground).
- Building a well-trodden application, like an AI ChatBot, assuming the company is willing to share its data.
On the flip side, open source models are based on transparency, offering more freedom and flexibility. And if your requirements fall outside the options above, it might be your only viable option.
As these models are developed in collaboration with the community, they tend to offer more permissible licenses. In some cases, they can even be run locally.
Therefore the development of open source models gives businesses an opportunity to adapt models where required and apply them in areas such as defence and life sciences, where, until recently, proprietary models were untenable in certain use cases.
The power of open source: Can they match GPT-4’s calibre?
In addition to providing more permissible models, the open source community may also be key in generating an alternative to GPT-4. At the time of writing, the top contenders are still a way off, but that could change in 2024. The benchmark scores have been slowly creeping up, and with the advent and spread of alternative training techniques an open source model on par with GPT-4 doesn’t seem too outlandish.
Moreover, the open source leaderboard shows an underlying theme that may be important. The top models are Mixture of Expert models (MoEs). These are a collection of expert models specialising at different tasks to make them overall generalists. MoE models can be more efficient than traditional LLMs, and the approach is a great way to scale model size while keeping the compute budget roughly constant. As a result, you can achieve better bang-for-buck in terms of pre-training loss performance.
In contrast, papers have shown that although MoEs perform better in pre-training, the improvement doesn’t always translate to stronger results on downstream benchmarks. For example, the Switch-MoE (a 1 trillion parameter Mixture-of-Experts from 2021) outperformed OpenAI’s GPT-3 on memorisation-based tasks, but not on reasoning-based ones. Such behaviour is not ideal for building trust with users as understanding model reasoning is crucial for transparency and validating that the model is aligned with human chain-of-thought.
As SLMs and RAGs evolve, use case applications will likely rise
2024 is likely the year of incremental gains and wider implementation of productionised systems. New models won’t bring real breakthroughs, and LLMs will remain intrinsically limited and prone to hallucinations. Yet, iterative improvements will make them “good enough” for various tasks, widening their usefulness.
Small Language Models (SLMs) are already being developed by Microsoft to help coders.
Cost-efficiency and sustainability considerations will only accelerate this trend. Quantisation, the process where models are compressed to reduce their memory footprint, will also improve, driving a major wave of on-device integration for consumer services.
The reduction in model size, the open sourcing of models, and their increase in performance will only help to aid the adoption of GenAI. Having smaller models enables companies to host models privately and therefore be safe in the knowledge that their data is not being sent to a third-party provider. Such changes enable more work to be done with sensitive data, expanding the application of GenAI to areas like healthcare and defence.
Likewise, improvements in retrieval augmented generation (RAG; where the LLM is exposed to highly specific data for more tailored responses), data curation, and better fine-tuning will increase the usefulness of LLMs for different applications. This will likely result in greater adoption across a wider variety of industries and services.
For instance, RAG, coupled with privately hosted small models, will enable businesses to produce chatbots to help them query sensitive data on premises. Moreover, pipelines utilising RAG, with its focus on privately held data, can help build trust in systems since models should, in theory, hallucinate less and be able to reference results based on the data they have access to.
The industry is also learning how to incorporate LLMs into their wider pipeline. As libraries like LangChain become more established and stable and the infrastructure around LLMs (e.g., vector stores) gets better, the risk of productionisation will decrease. This means that 2024 will likely see more production-based work than experimental POCs. As a result, 2024 could mark a major milestone in the use of LLMs across every industry.
Start building your Generative AI Roadmap today
At Faculty our Generative AI Roadmap helps you to identify the genuine opportunities that GenAI can tackle within your organisation, both today and in the future. Get an actionable plan to build the capabilities you need to outpace your competition. Click here to find out more.