Blog
How to make successful investments in AI: Start with the problem, not the data
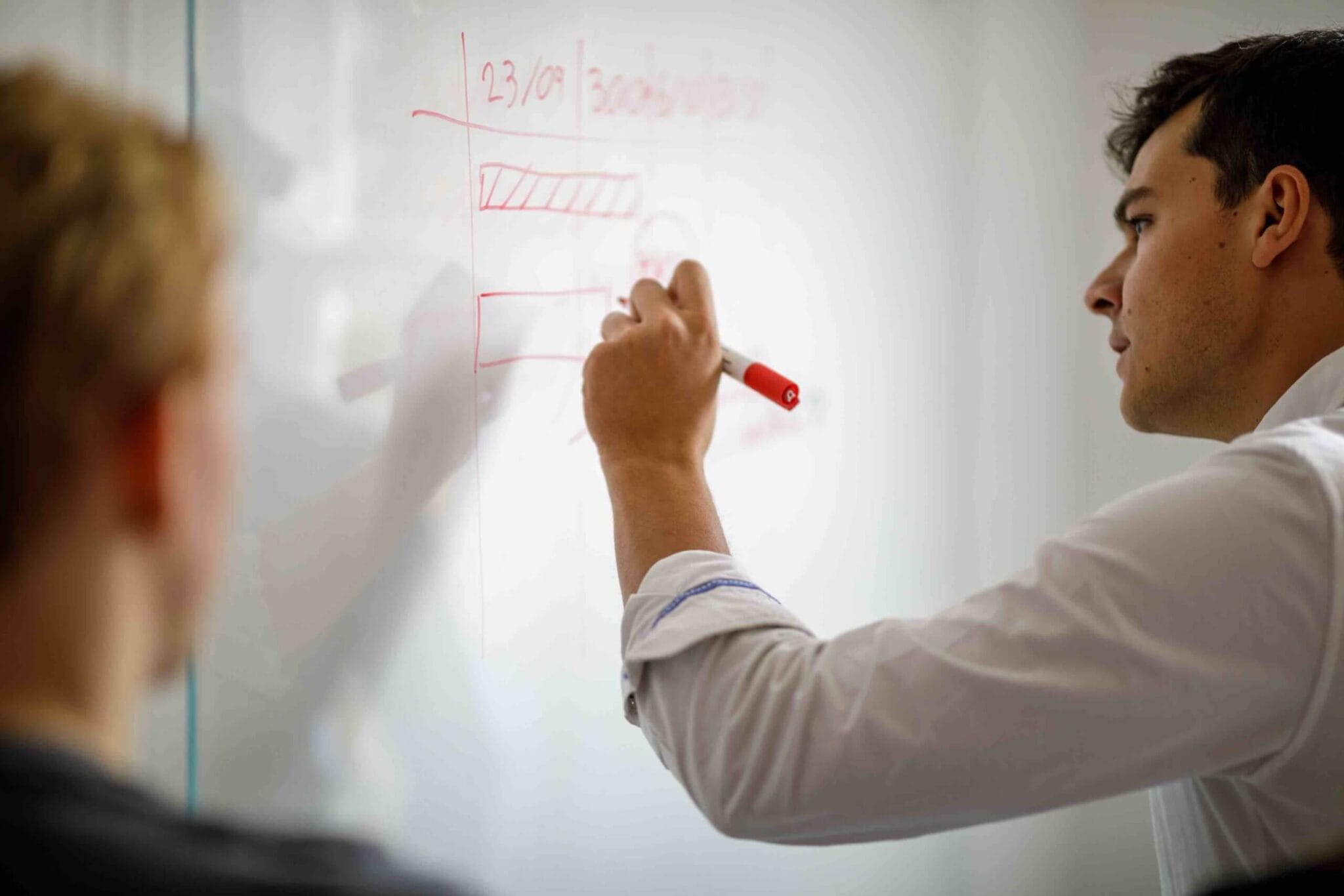
At almost every single conference that I go to, I hear how: ‘the world’s most valuable resource is no longer oil, but data’. Speakers often reel off how Alphabet, Amazon, Apple, Facebook and Microsoft are bathing in cash, and the reason behind this is because of the sheer amount of data that they collect.
This obsession with data is also on display when we speak to some organisations. I have been involved in numerous conversations with frustrated CTOs / CDOs who talk about how they have spent years building their data infrastructure, often investing millions of pounds in the process but struggled to generate much value from it.
While data is an asset, it’s also a red herring. By focusing their attention on data, organisations have frequently ignored the first (and most important) part of the puzzle: the opportunity.
I’m going to give you a quick overview of Faculty’s approach that is designed to help organisations identify the right opportunities to extract value from your data. This is a framework that we have applied to a wide variety of organisations; from venture-backed start-ups building their business on AI, to large FTSE-100 enterprises and most recently, the UK government.
Regardless of the size or sector, it is designed to help ensure that you; a) pick the ‘right’ opportunities now; and b) focus your attention on what you need to do in the future.
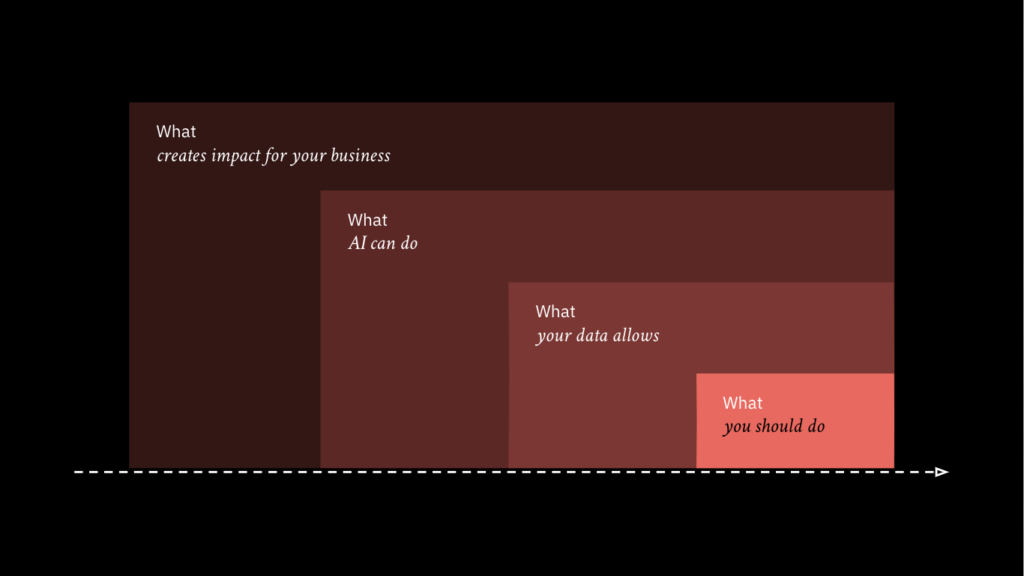
What creates impact for your business
Data itself is only useful when it is linked to an opportunity or business problem. Data science is only useful when it results in the proverbial ‘actionable insight’. In order for something to be useful in the real world – to make AI real – you have to start with answering ‘what creates impact for your business’.
By addressing this point upfront (rather than messing around with the data), you can begin to build a picture of what the biggest opportunity areas are in your business, and start your journey towards extracting real value from your data.
Getting to this stage practically involves a lot of structured workshops, interviews and conversations with key stakeholders in the organisation. The output of these being the identification of a ‘longlist’ of projects, with varied levels of complexity and impact, that will form the basis for further exploration.
What AI can do
The second point is harder to address, as it requires specialist domain knowledge of what this technology can do. In this phase, we need to take the longlist and try and identify which of these opportunities could be solved using AI; the output being some sort of ‘medium list’ for you to focus your attention on.
In identifying what AI can do, it’s sometimes helpful to ask the question; ‘could a human, in principle, do this task?’. While good AI opportunities are normally those that predict things, recommend things, categorise things, convert speech to text, or identify things (eg computer vision); if it is unlikely that a human could infer the necessary information from a dataset, the chances of an AI-led approach succeeding are significantly lower. For example, if a human finds it difficult to identify whether the phrase: ‘that’s sick!’, is either a good thing or a bad thing (it depends on your age, trust me) – AI will not be able to either.
When addressing this point, it’s also useful to spend some time doing some research to see if there are examples of this particular opportunity being solved elsewhere. This will help determine; a) whether this opportunity can be solved using AI; and b) potentially how successful you can expect to be.
It’s also entirely possible that research into ‘what AI can do’ will result in the identification of some exciting new opportunities to add to the list (particularly if you have knowledge of the latest R&D developments). AI is a technology that enables new projects that were not possible before – so be prepared to add to your ‘medium list’!
This phase often fails if a data scientist is not involved, and / or if the non-technical team is not trained in the basics of data science. If you are lacking in either of these critical skill sets, you may need to either: bring in a specialist to help you; or hire new / upskill existing employees to fill your skills gap.
What your data allows
Now we have our list of potential AI opportunities, we can delve into the data and get our hands dirty.
Here we must start by mapping the different datasets available to each of the opportunities previously identified. Once we have done this, we can then assess to what extent the data supports the opportunity. Typically this involves a data scientist analysing the data for things like:
Accessibility – how quickly can the data be accessed and by what means (eg batch upload, API etc.)?
Frequency – how frequently is the data updated and how does this impact the use case in question?
Completeness – how many missing values exist within the data and are they consequential for the use case?
Structure – what format is the data in and does it require significant preparation to be ready for modelling?
Linkage – can we link key variables within and between datasets? Do unique identifiers exist?
This analysis will allow you to build up a picture of what your data allows, ultimately informing the creation of an opportunity shortlist.
As a result of this exercise, you will also be able to see where your data falls short; meaning you can now specifically target any remedial activities / additional datasets required to unlock further value in the future (instead of blindly ingesting data into a humongous database).
What you should do
The last hurdle is all about taking this shortlist and identifying ‘what you should do’. Practically this means ranking the opportunities based on your strategic priorities; therefore giving you a clear starting point for you to begin extracting value from your data.
There is no uniform answer to this question, as it differs from organisation to organisation.
Some organisations want to identify some ‘quick wins’, those opportunities that have high impact but low technical complexity, in order to help build momentum. Others want to push the frontier and focus on the most innovative applications of AI, perhaps to support the launch of new business or proposition. Some may want to reduce costs through the automation of cumbersome processes, some may want to boost revenue by increasing sales and efficiency.
Whatever your overall strategic goals are, by the end of this phase you would have hopefully picked the right opportunities that deliver on them.
As a final point, it is important to say that these roadmaps are not fixed. Data science is all about learning and experimenting. Your opportunity list should (and will) change as you build your first models and learn / unlearn things about your projects. This is why we always try to tie our strategy projects to the practical execution of prototypes, to try and prove / disprove hypotheses early doors to de-risk investment.
And that’s it! A (very) quick overview of a framework that we have developed over the course of successfully completing over 350 data science projects.
If you would be interested in finding out more about this, and how we might be able to help you on your AI journey, get in touch!