Blog
What is AI Safety? Towards a better understanding
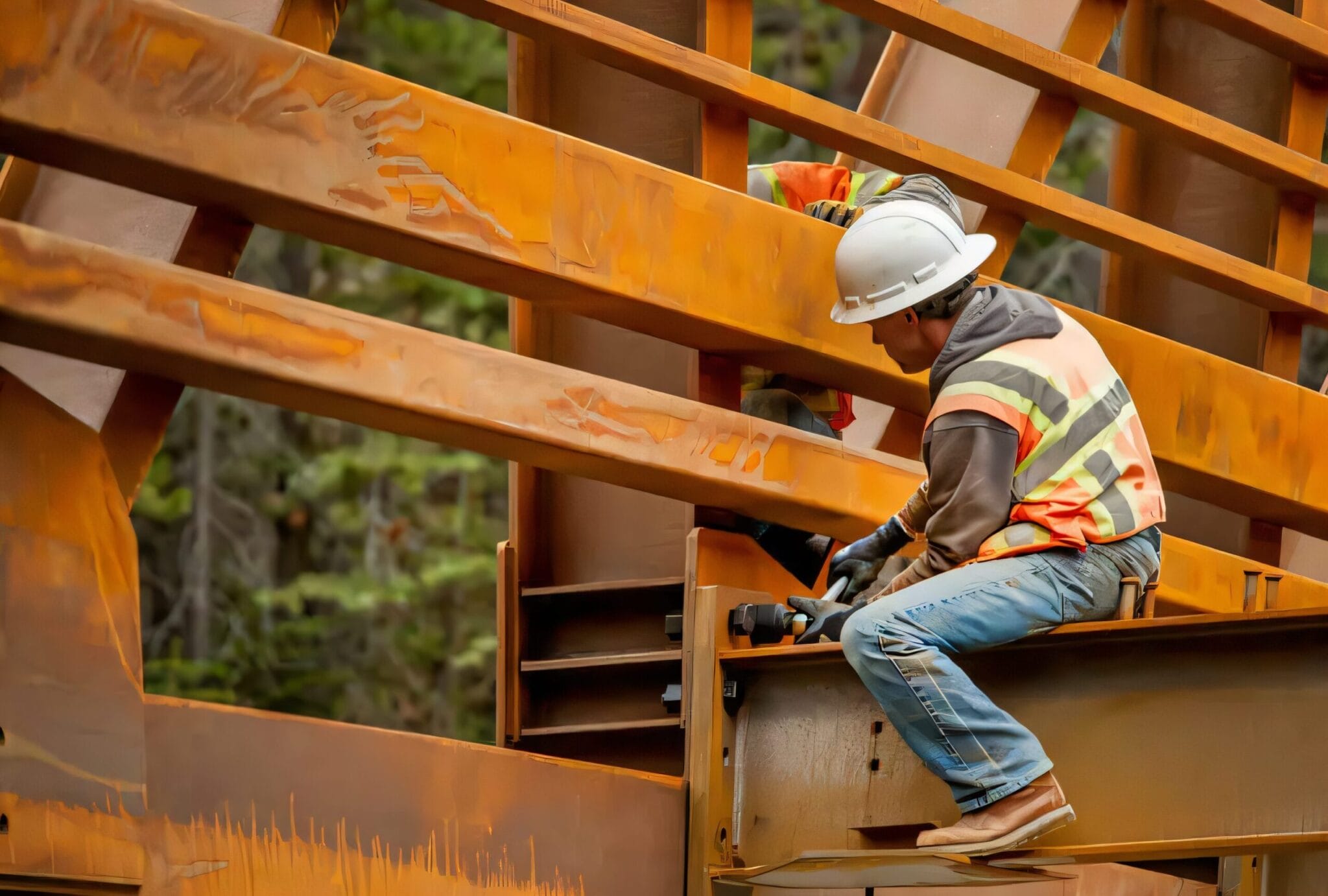
Table of Contents
Artificial Intelligence Safety (AI Safety) can be broadly defined as the endeavour to ensure that AI is deployed in ways that do not harm humanity.
This definition is easy to agree with, but what does it actually mean? Well, to complement the many ways that AI can better human lives, there are unfortunately many ways that AI can cause harm. In this blog, we attempt to describe the space of AI Safety; namely, all the things that someone might mean when they say ‘AI Safety’.
AI Safety space
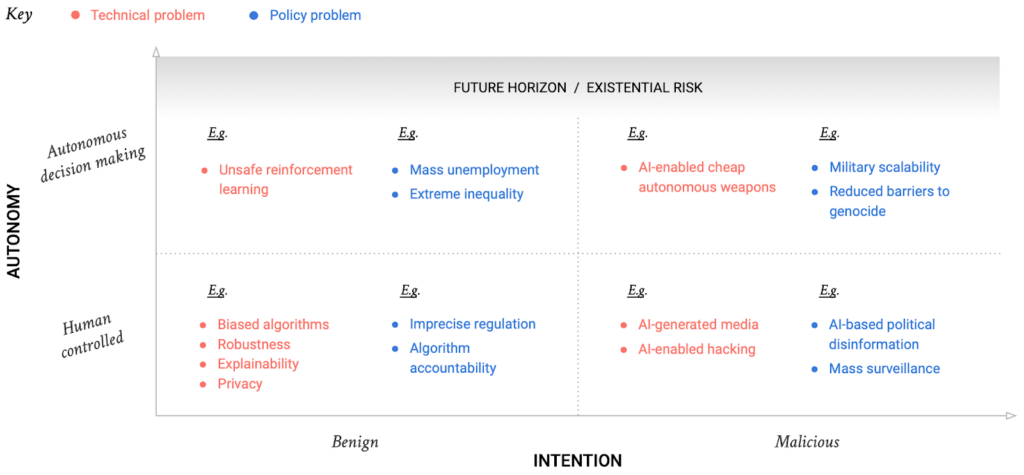
Three quadrants where we think technical efforts should be focused
1. Autonomous learning, benign intent
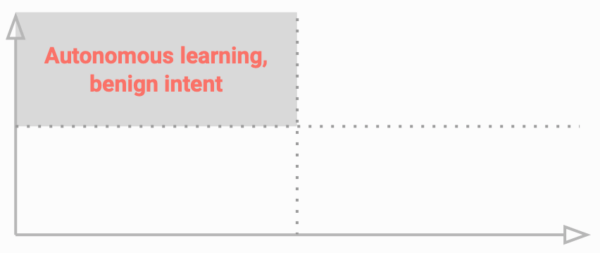
Autonomous learning agents learn as they go, which means it’s much harder to know exactly how they will behave when deployed. Basic problems, such as how interrupting such agents can effect how they learn to behave, or how the absence of a supervisor can affect the actions they choose to take, are unsolved in most cases. Furthermore, these agents can unsafely explore their environments, hack their reward functions and induce negative side effects, all in an attempt to do what they were built to do.
These problems are naturally formulated in the context of reinforcement learning, and are discussed in more depth here. We have recently developed an algorithm that addresses these problems, enabling safe reinforcement learning in contexts where humans understand safe and dangerous behaviours. Our recent paper exploring this subject further can be found here.
There are also major policy questions associated with autonomous learning agents, especially as a consequence of their ability to automate tasks that were traditionally achievable only by humans. As a result, sophisticated autonomous agents could lead to large-scale unemployment and inequality. Understanding the impact of this, and mitigating the downsides while preserving the benefits as much as possible, will be an ongoing policy challenge.
2. Human controlled, benign intent
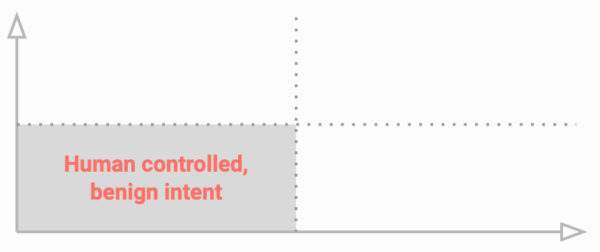
Examples in this bucket include supervised algorithms like classifiers that are trained, frozen into code and then deployed to make useful business decisions.
Despite the simplicity and the benign intent, AI Safety concerns still arise. Even if the algorithm is shown to perform well on a held-out test set of data, it might not work as intended for the following reasons:
- Non-robust: sophisticated AI algorithms can be non-robust in the sense that, despite performing well on their test data, they behave very differently on other data (either similar to the test-set data in the case of adversarial examples, or different from the test-set data in the case of distributional shift).
- Biased: well-trained, robust algorithms can still reflect biases that exist in the training data or that arise in feature selection. Identifying and preventing the potential harm of these biases is a challenging task.
- Privacy violating: if the deployment of an algorithm exposes information about members of the training set, sensitive information can be gleaned directly from the model even without the release of training data.
- An algorithm that does not suffer from any of the above problems may be a trustworthy algorithm, but it is not necessarily an easily interpretable algorithm. The lack of explainability of AI algorithms presents a problem of its own by making it difficult to conduct an external audit, as well as by frustrating one’s ability to provide a reason for any decisions made by the algorithm.
We have so far mentioned only technical aspects of AI algorithms in this quadrant. There are also policy considerations, such as assigning individual human accountability for the safety of an AI algorithm. This is a non-trivial challenge, since there are many contributors to the libraries used to develop the algorithm, as well as the data scientists who created the algorithm, the engineers who deployed it, the team lead who gave the okay, and on up to the CEO of the company deploying the algorithm.
3. Human controlled, malicious intent
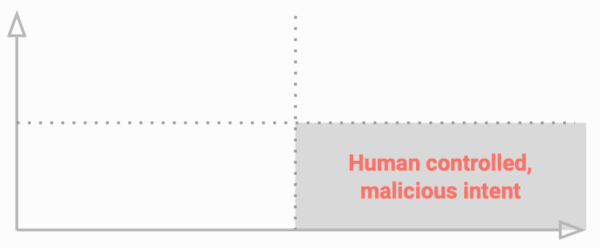
Mitigating the unintended consequences of deployed AI algorithms is not the only concern to consider. There will of course be malicious usage of AI that needs assessment and counter-strategies.
Many of these arise from the ability of AI algorithms to process unstructured data, enabling them to recognise faces in images and even to generate new images of a person’s face or new audio of a person’s voice. As a result, AI makes it possible for highly effective mass surveillance, which could be used by a state or organisation to exert unprecedented control over a population. Similarly, the ability of AI to generate faces and voices allows the creation of high-fidelity disinformation, used either for individual defamation or for political means (e.g. fake news).
The solutions to these problems are likely to be both technical and policy-based. We are creating detection technology that can classify video footage as ‘real’ or ‘fake’ as a first line of defence against AI-based media disinformation. We are similarly creating educational content to show the power of these technologies as an ‘inoculation’ strategy. However, on the surveillance side the required technology already exists, and could only be mitigated by individuals obscuring their faces. Therefore, the extent to which organisations are allowed to collect data on individuals at scale based on facial recognition is a pressing policy consideration.
Investing in AI? Want to maximise its value? Contact our team today.