Blog
Generative AI: how to cut through the noise and identify where it can make the biggest impact
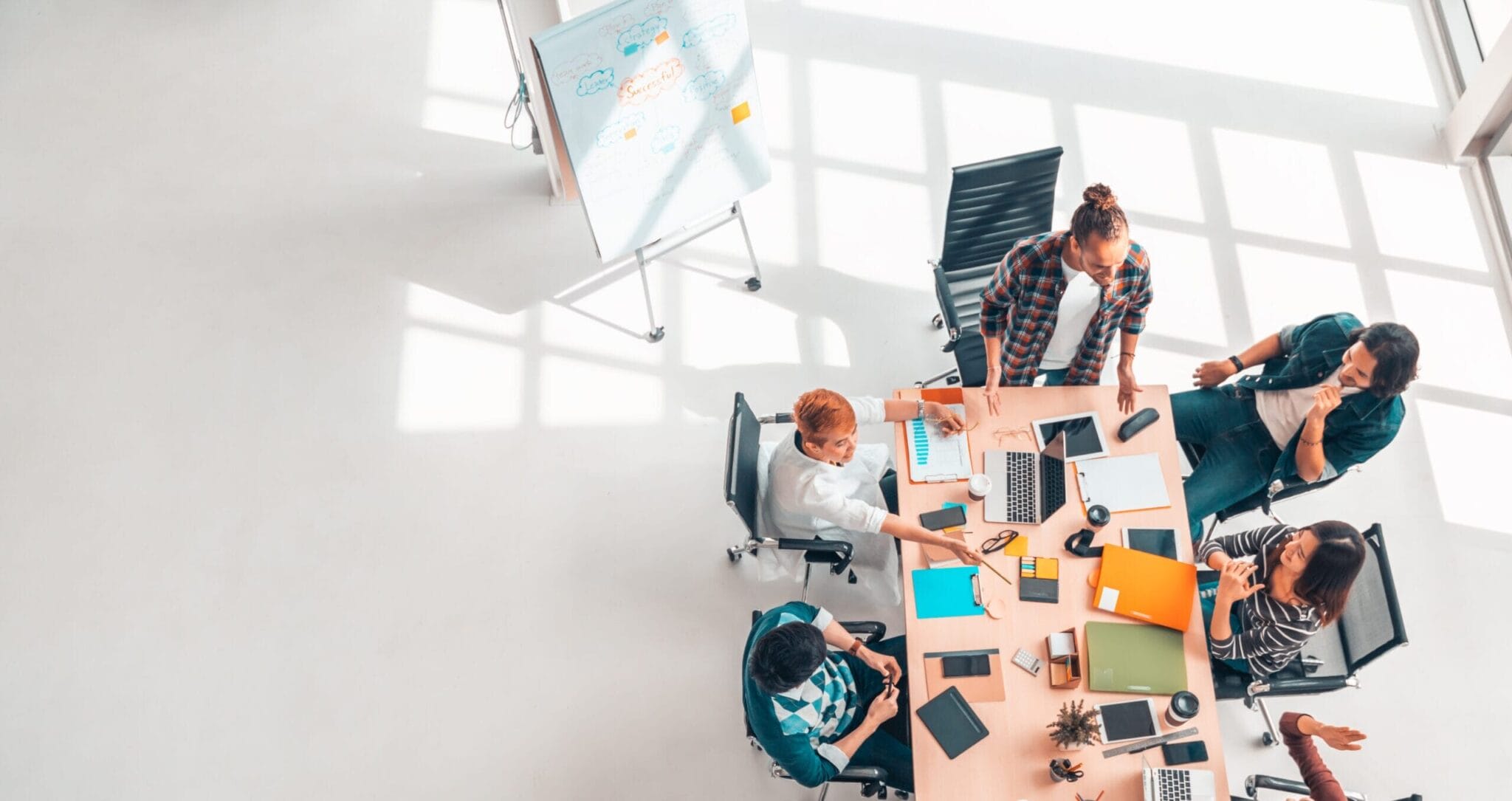
Since the launch of ChatGPT in November 2022, Generative artificial intelligence (GenAI) has become a hot topic of conversation in boardrooms. It is obvious to organisations that the change it is bringing will fundamentally alter business models and markets. Nevertheless, for many, a big question remains: how do you pinpoint where GenAI can have the biggest impact on your business? To answer this question, it is important to understand not only the potential of GenAI but also the problems, priorities, and goals you are trying to achieve.
When we see rapid technology shifts like GenAI, there is a tendency for organisations to myopically focus on the technology itself rather than looking at it through the lens of how it can solve problems and align with business goals. Remember when every company needed a metaverse and blockchain strategy? Now the focus has shifted to “we need to develop a GenAI strategy-and fast!” This can result in forward thinking executives roaming the hallways looking for text summarisation problems to solve, and rushing to integrate chatbot UIs into every process.
While at first glance, these experiments can produce some impressive looking proof of concepts, more often than not, they lead to fragmented and disconnected GenAI pockets across an organisation that are not properly integrated into operational processes and therefore deliver little to no tangible value.
In contrast, the organisations making the fastest progress remain focussed on the problems that matter most, and only get excited about GenAI when it is a good fit for solving them.
Successfully Scoping Transformative GenAI
At Faculty, we help clients successfully scope and deliver transformative GenAI solutions across a wide range of industries, including healthcare, retail, and finance. After a decade of learning and experience, we have refined our approach using tried and tested methods. So if you’re trying to figure out where to begin or how to prioritise opportunities when it comes to integrating GenAI into your strategy, here are some of our key insights to guide you on the right path:
1. Start with education
Ensure executives have a common understanding of GenAI. Foster alignment and buy-in on opportunities and focus areas, and enable top-down alignment of transformation priorities.
2. Focus on processes rather than individual ‘use cases’
Take one of your core business processes as the focal point, aiming to transform it end-to-end. Ideally, this process is a key driver of performance and has applicability across the organisation.
- While some organisations may wish to run through an analytical exercise to assess and prioritise the right business process, in our experience, practical factors often override overly analytical decisions. We suggest choosing a process that has a strong business case and stakeholders ready to support its implementation.
- Let’s take the example of a telecoms company whose priority is to improve customer satisfaction (CSAT) scores. Given interactions with customers take place via natural language, it would be easy to quickly identify a variety of GenAI use cases that could feasibly improve CSAT, such as copilots to support customer service agents or customer-facing chatbots for the website. However, this approach risks resulting in a series of disconnected POCs. To drive significant performance benefits, focus should be on transforming the end-to-end customer service process through technology.
3. Break down the process into tasks
Once the priority process has been identified, you need to determine which steps in the process should be optimised first. We suggest breaking down the end-to-end process into a modular series of connected tasks and then categorising these tasks according to their contribution to the priority business objectives.
For example:
- Time/cost to complete
- Business value they generate
- Risks associated with not acting, etc
Having identified the priority tasks, you can then focus on exploring use cases to improve the performance of each individual task, and then using AI to connect them back together to create a new optimised end-to-end process flow.
For this approach to work well, it is key to have a cross-functional group of senior executives who understand the big picture, business process owners who understand the intricacies of the chosen process, and AI translators who understand the business and the capabilities and limitations of the technology.
4. Talk only about commercial value
Your vision for AI-enabled end-to-end transformation should be framed solely in terms of the commercial goals and metrics that matter most to your business. By identifying the key drivers of those metrics and quantifying the contribution that each individual use case makes to driving the overall commercial goal, you can make data-driven decisions around use case prioritisation and sequencing. An obsessive focus on commercial outcomes ensures your AI transformation doesn’t fall into the trap of becoming yet another technology vanity project.
5. Build common capabilities
While the initial priority use cases should all have a standalone business case, they should also contribute to building foundational technology capabilities that can be reused to accelerate future use cases.
For example, many of your use cases will likely require the ability to search and store documents to provide context to the Large Language Model (LLM) via retrieval augmented generation (RAG). The core architectural components of any RAG system is an embedding service to translate text into machine-readable embeddings and a vector database to store and search those embeddings. By developing this core capability early in your journey, it can then be reused over and over again for a variety of use cases with marginal incremental effort.
Start building your Generative AI Roadmap today
Ready to unlock the full potential of GenAI for your business? Click here to reach out to our team today to explore how we can help you tailor it to fit your business needs and goals.