Blog
Generative AI for industrial operations: 3 ways to get started
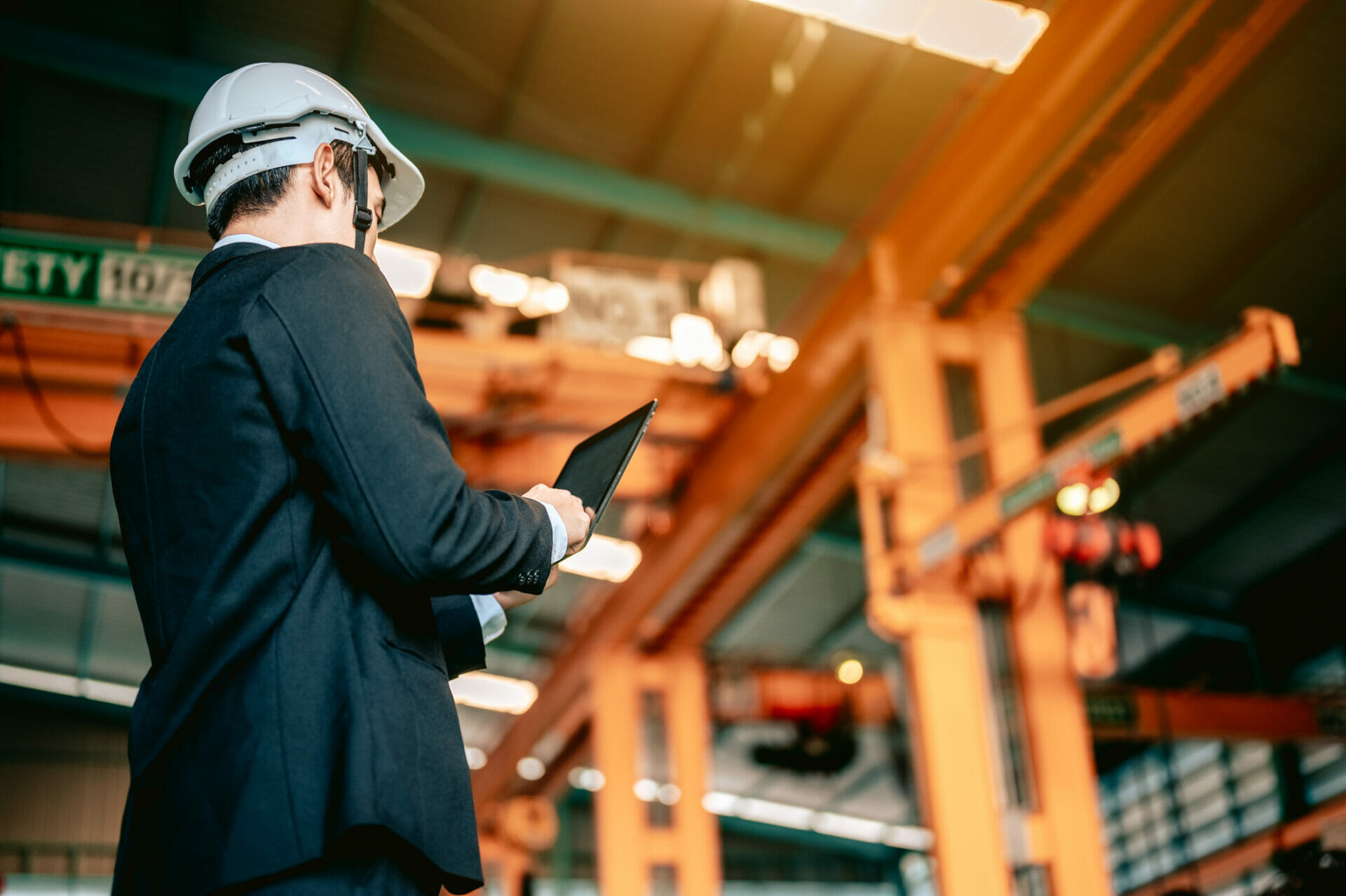
For companies operating in the B2B industrial sectors, the impact of generative AI may feel remote at first glance. But ignoring its potential for supercharging your operations would be a significant oversight.
Large language models (LLMs) – the tech behind ChatGPT from our partners OpenAI – have grabbed many headlines of late for their leaps forward in content creation, AI assistants, and a host of other consumer-facing applications.
But there are also many compelling use cases for LLMs in the world of operations and maintenance (O&M). And when applied to the right problems with the right expertise, the results can be transformative.
Here are three starter use cases for applying LLMs in your O&M.
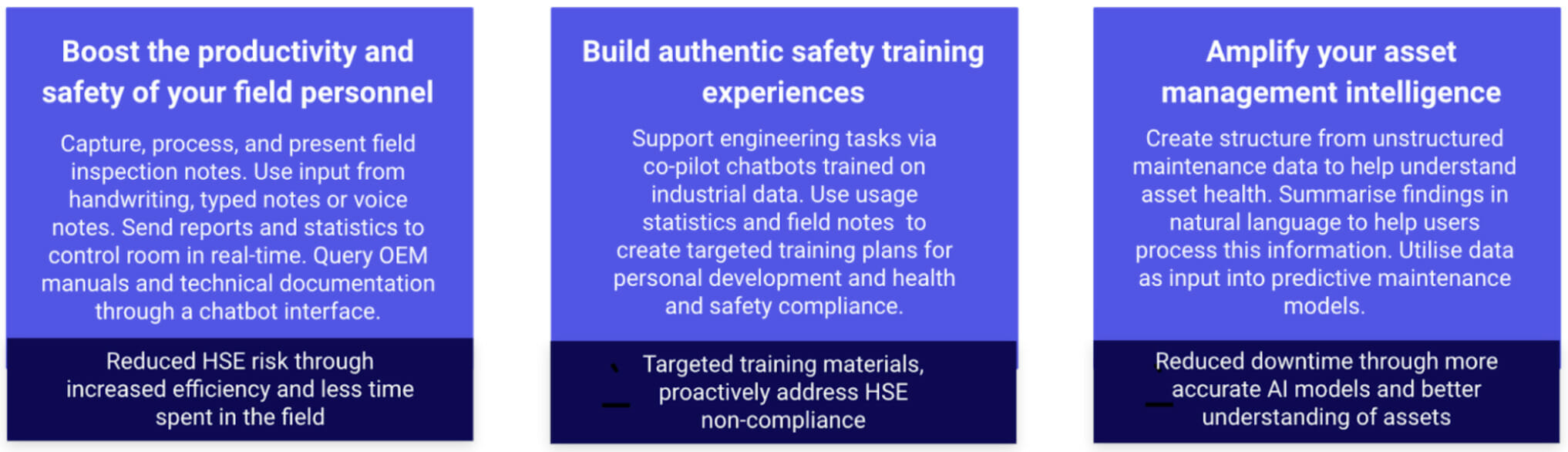
1. Boost the productivity and safety of your field personnel
Manually completing and analysing field inspection notes can be hugely time-consuming. Using LLMs for innovative applications can free up your engineers’ time for value-adding operational tasks. And reduce their HSE exposure by reducing their time spent in the field.
For example, have your engineers voice record their progress while performing their tasks. Passing these recordings through a speech recognition model will generate an accurate transcript by the time they finish.
At Faculty, we helped a leading transport company improve its safety-critical communications using an Automatic Speech Recognition (ASR) model for this kind of auto transcription. The solution achieved a word error rate of less than 1%, compared to a typical 4-5% if done by a human or alternative ML models.
Feeding this transcript data into an LLM solution can automate the completion of your engineers’ reporting forms. And draw out actionable insights for the control room or corporate-level reporting.
Generative AI can also help your team to navigate relevant industrial data about a component or asset. Field engineers will frequently need to query guidelines and data such as P&IDs, technical documentation, OEM manuals and work orders.
A custom-made generative AI solution with a chatbot-style interface can be a powerful way for an engineer to surface the relevant material while out in the field, without having to manually search the original documentation.
A sufficiently trained LLM could even summarise exceptionally technical diagrams and documentation. We know from first-hand experience that this kind of work requires a lot of training time, but the potential is there if handled in a responsible, safe way.
2. Build authentic safety training experiences
Safety and compliance training for staff is essential for any responsible operator. Generative AI can help you create a tailor-made training programme enriched by your own team’s performance data.
Feeding the recorded data of your operational teams’ communications into an LLM can help identify their strengths and shortcomings with HSE or operational protocols.
Returning to the transport company example above – we fed transcripts of communications between front-line personnel and their control centre into a custom LLM. This generated accurate summaries and quantitative assessments of safety performance.
The solution scrutinised the data in a fraction of the time a human would take. It also confirmed that AI could complement the monitoring and evaluation of safety-critical comms in real-world situations. The scale of data collected has also enabled the company to drive better-targeted safety training for staff.
A training solution powered by generative AI can use real cases in this way to produce more authentic and customised scenario training materials to meet your training goals.
3. Amplify your asset management intelligence
Traditional machine learning techniques rely on working with structured data, typically stored in structured databases. But one of the key strengths of LLMs is working well with unstructured data which may otherwise go underused.
For instance, the unstructured maintenance data in your field engineers’ notes and communications can be a treasure trove of operational insights. The kind that can reveal the health of your components and assets, providing ideal material for training machine learning models to identify where failure events may occur in the future.
This rich information can supplement traditional telematics and Industry 4.0 datasets to feed into your prediction models in a way that hasn’t been possible before.
Taking the ‘art of the possible’ even further, generative AI solutions such as LLMs can be ideal for creating synthetic data. A sufficiently-trained LLM can use your field engineers’ reports as source material to produce many more synthetic versions.
In doing so, you can vastly increase the volume of training or testing data available for your prediction models. The result is more reliable predictions for both unusual events and the more likely outcomes around the lifecycle of your assets.
This is just the starting point for LLMs
Generative AI and LLMs are evolving rapidly, and with them, the potential use cases in B2B industrial O&M will continue to multiply.
There are a number of factors to consider to make sure your generative AI-powered solution provides lasting and reliable value. But when these are navigated properly, the possibilities are compelling.
At Faculty, we’ve helped hundreds of businesses assess, tune and integrate AI products into their wider processes and systems. Our CCO, John Gibson, has outlined the fundamentals in more detail in his guide to generative AI for organisations here.
How could generative AI give a shot in the arm to your O&M processes? Get in touch for a live demo of these uses cases in action.