Blog
Digital twins in the energy sector: transforming hype into action
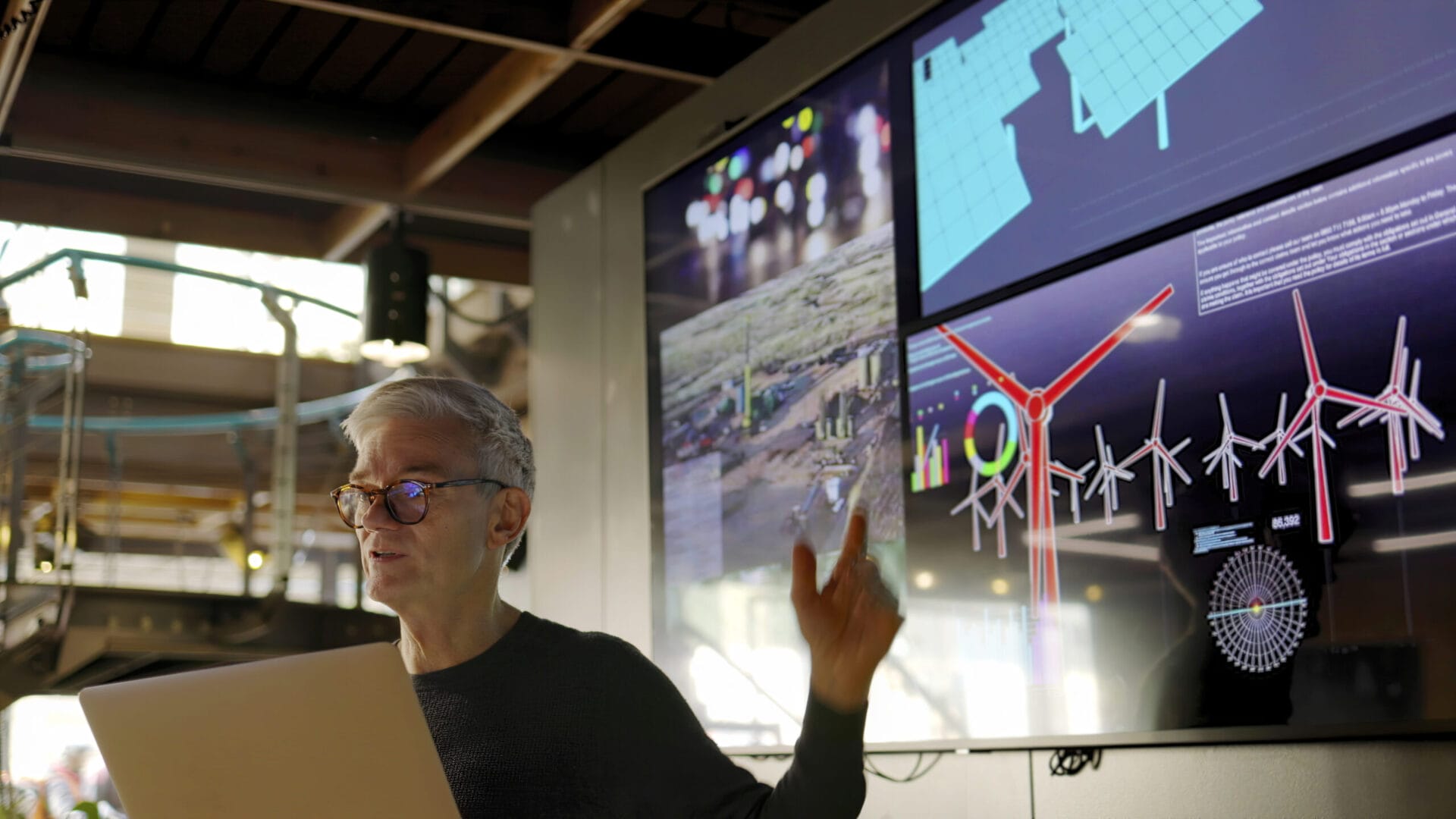
Digital twin (DT) technology is enhancing performance, safety, and decision-making across a wide range of industries, from manufacturing to construction to healthcare. Yet, despite much hype about its potential, adoption in the energy sector remains slow, with no clear roadmap for success. So, how can the energy sector overcome these obstacles to reap the benefits? Let’s take a closer look.
What is a digital twin?
A Digital Twin (DT) is often defined in different ways by different organisations and industries. But in its simplest form, it is a digital copy of a physical asset, system, or process. Digital twins allow you to create a detailed replica (using real-time data) of the real thing to test, optimise, and plan more efficiently without compromising safety, security, or stability.
For instance, a digital twin could be used to test the strength and resilience of a new bridge before any cars travel on it, or to assess the environmental impact of a new office building before it goes up.
There are four main types of DTs in the energy sector, each with a set of different capabilities:
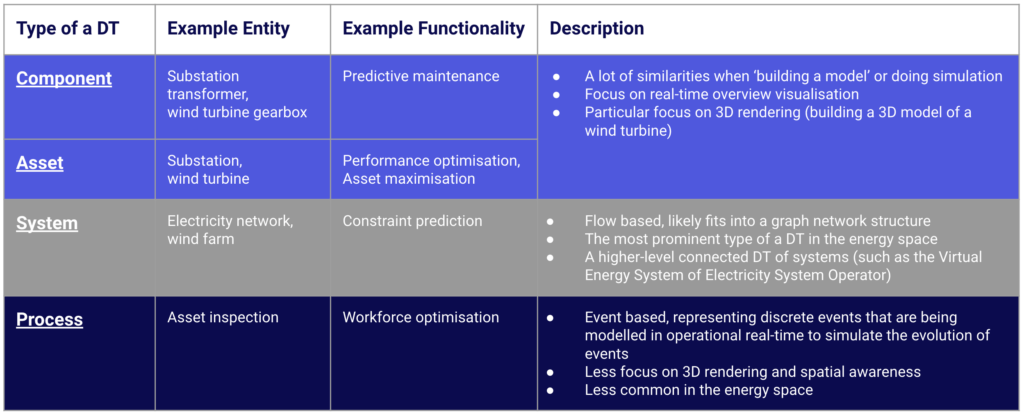
Energy companies can also build and utilise a network of DTs reflecting real-world elements, such as power plants, grids, wind turbines, and other related infrastructure
The concept is especially relevant to energy networks, where the term ‘interconnected’ DT refers to multiple assets or components owned or operated by different bodies but integrated into one DT model.
To build a DT network like this requires the use of data across multiple systems. This allows organisations to gain a deeper understanding of how all elements interact instead of just examining one asset in isolation.
Popular use cases in the energy sector
There are endless possibilities for using digital twin technology in the energy sector. For instance, a DT could be used to create digital replicas of power plants to identify bottlenecks and take proactive measures to prevent future problems. Or they could be used to model and assess the efficiency and performance of wind turbines remotely without actually going near one.
Here is an overview of some of the most common capabilities of DTs in the energy space:
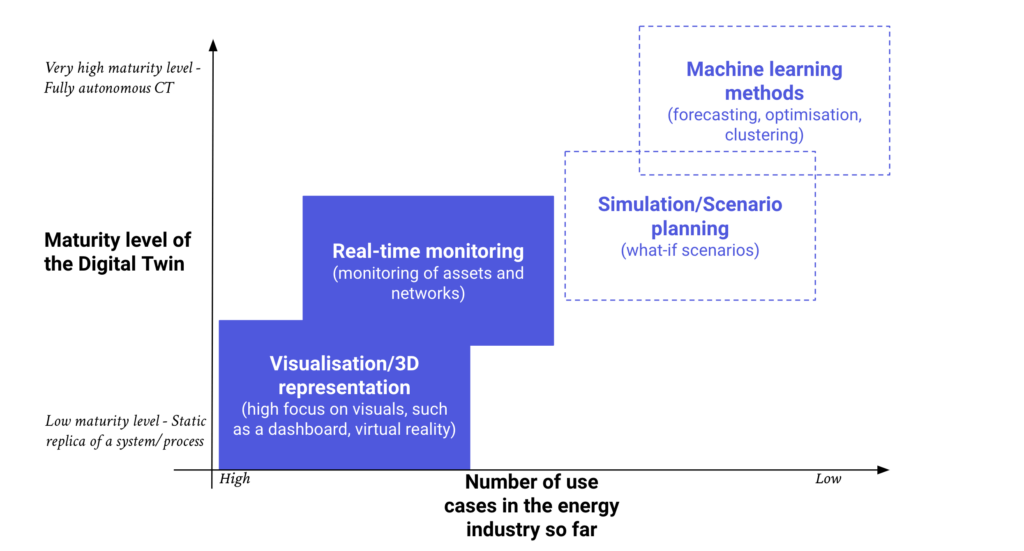
Digital twins fuelled by AI and machine learning
In the energy sector, most DTs are mainly used for visualisation and monitoring in real-time and are just starting to explore the full potential of DTs through AI and machine learning. In contrast, industries such as aerospace, manufacturing, and automotive are using DTs in a more sophisticated way, for example using predictive analytics or simulating the production phase.
With our help, companies can benefit from the capabilities of these more advanced DTs with AI built-in, gaining deeper insights into predictions, scenario planning, and optimisation.
Benefits for the energy sector
Energy, like most other sectors, faces a growing need to transform its operations to stay competitive. As a result of climate commitments and the need for cleaner and more sustainable fuel sources, change is now at the top of every company’s agenda.
Digital twins offer the sector an efficient and safe way to explore new possibilities without disrupting existing systems. This route offers many benefits for the sector, but arguably, consumers stand to gain most from the more cost-effective and environmentally friendly options digital twins can bring to the table.
Benefits for the sector include:
- Improved performance and more predictable results – DTs enable real-time and continuous monitoring of assets, processes, and systems to ensure everything is running as efficiently and effectively as possible.
- Ability to spot and solve problems early – DTs allow you to analyse data continuously and apply predictive models to anticipate problems before they occur.
- Greater efficiency leading to reduced costs – instead of building costly prototypes, using a network of DTs can give you a complete picture of an entire energy ecosystem so that you can plan more effectively and identify key areas for improvement.
- Risk-free experimentation and testing – by simulating and modelling different scenarios, DTs allow you to test and optimise strategies without compromising the real infrastructure.
- More informed decision making – DTs can be used to help you understand how changes could affect an asset, process, or system’s overall performance. Allowing energy companies to plan for the future and make better decisions.
In order to take full advantage of DT’s capabilities, a company must first define the right use case, which can vary depending on its requirements, the problem it wants to solve, and the industry sector it operates in.
Moving from hype to action
In light of the endless possibilities and many benefits DTs can offer, why are they not being used more readily in the sector? The truth is that creating DTs that consistently deliver value and reliable results over time is easier said than done. The process takes a lot of careful planning and expertise.
Many obstacles still stand in the way of wide-scale implementation in the sector, including ageing infrastructure, lack of expertise, regulatory challenges, upfront investment concerns, on top of the vast amount of data analysis involved in making it happen.
However, with the right expertise in your corner, it is possible to overcome these issues and implement a successful DT strategy.
Here are some of our top tips for getting started:
Focus on the problem you want to solve and go from there
Is there a challenge you need to solve, or specific inefficiencies you need to address? Defining exactly what you need the DT to achieve, whether it’s to optimise the performance of a new system or predict potential problems in an older system, will increase your chances of success.
Use AI to take your data to the next level
Any digital twin is only as smart as the data used to develop it. By leveraging data from multiple sources and applying advanced analytics and machine learning algorithms within your DT, you will be able to extract valuable insights to maximise efficiency at every level.
It takes a village to create a digital twin
An effective DT requires input from across an organisation or industry. Involving as many interested parties as possible during the requirements gathering, development, and design process is crucial.
Continual improvement is part of the process
The process of creating a DT is not a one-time event, and you will not see meaningful results overnight. A successful DT requires ongoing monitoring, tweaking, enhancing, and optimising to ensure it is working as intended. The more time you invest upfront, the better the long term results.
Contact us to learn more about how digital twins can help your energy business. Let’s explore their potential together.
Co-Authors:
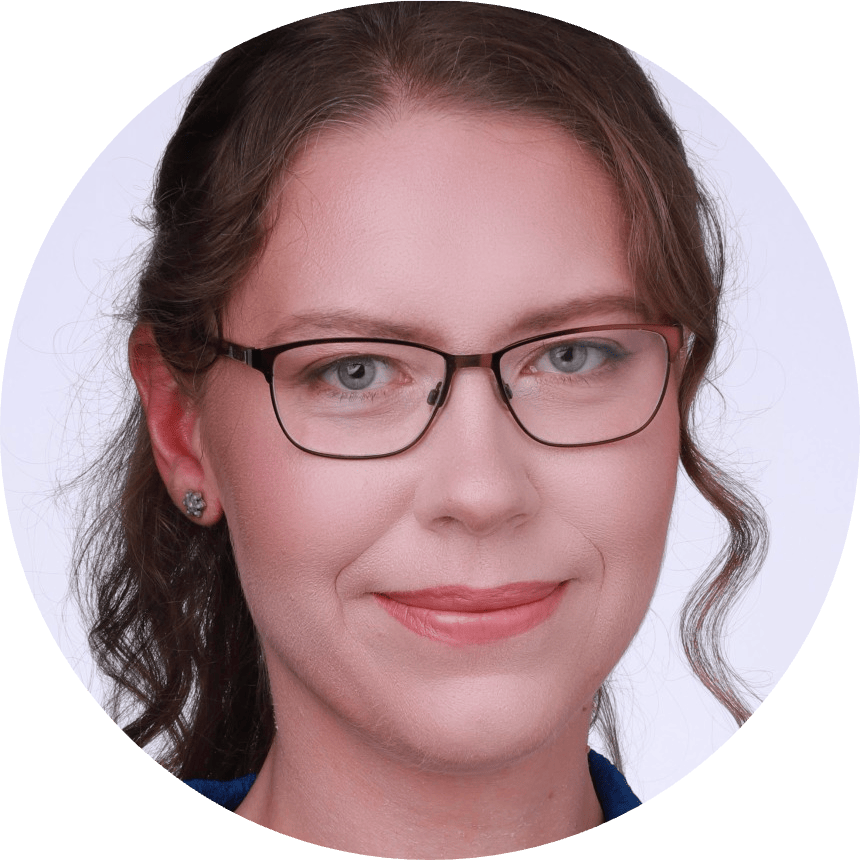
Katrina Soderquest
Senior Data Scientist, Energy Transition and Environment
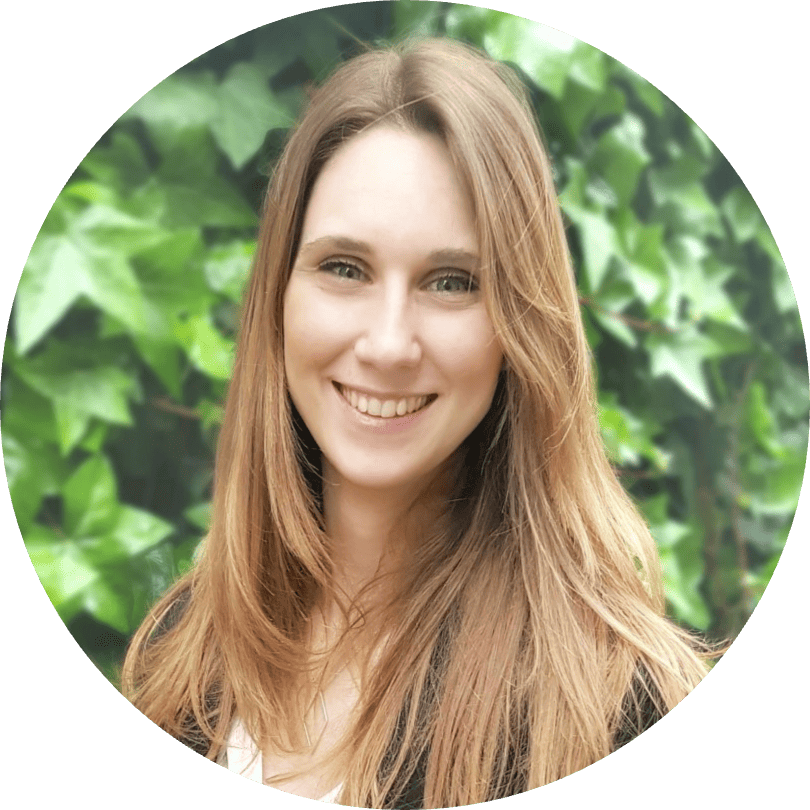
Júlia Sala-Bayo
Data Scientist, Energy Transition and Environment